The current boom in Artificial Intelligence raises questions about the ethics in that field. Many guidelines have been proposed to align the development with ethical principles, but the influence on the decision-making in the field is minimal and many companies like for example Google demand concrete laws. Those guidelines are not only concerned with the correct ethical decision of a agent, but also with privacy, accountability, explainability, fairness and other aspects that need to be addressed (Hagendorff, 2019).
The implementation of those principles is a challenge in the field because algorithms and data often lack some of these features. For other aspects we do not even have a clear definition or clear understanding of how we could implement them. One of those, as it is the case for autonomous vehicles, is how agents should act ethically if there is the possibility to harm people. There are different normative ethical theories, that can be considered in the design of agents. The consequentialist view states, that an action should have the most moral outcome. The deontological view says that the actions itself should follow some rules and the virtue ethics suggests that an action should follow some specific moral values (character traits).
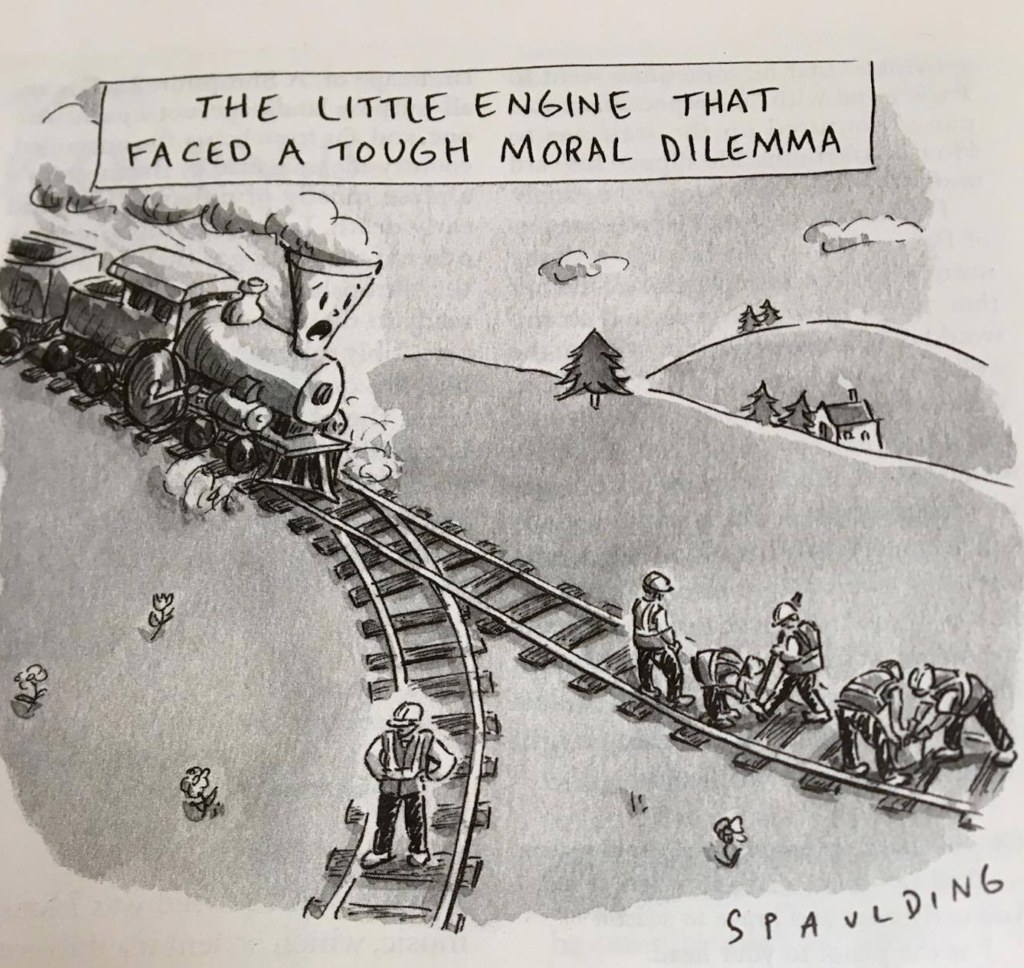
Many discussions nowadays are framed around the so called trolley cases, which are central in the ethical framework for autonomous cars. Here the question is whether a car stuck in a unavoidable situation should take action to kill just one person instead of more people or sacrifice the passenger to save other lives (Himmelreich, 2018). Even if those questions are relevant as an experimental paradigm it does not take into consideration all the mundane actions a car has to take. Already today self-driving cars are in many situations safer compared to a one steered by a human, mostly because the majority of traffic accidents are due to human error (Trubia et al., 2017). However, the focus on the trolley cases inhibits the development and concentrates too heavily on outlier cases, which can make a moral agent act insufficiently in more general cases. This view corresponds with the legal maxim that „Hard cases make bad law“.
There are two major ways of implementing ethical principles into agents. First, in a rule based manner called top-down, some moral principles are used (e.g. Kant’s categorical imperative or Asimov’s law of robotics). The second way is to treat the human as an ethical reference. A machine should learn how to act by observing the actions of humans, which is called bottom-up. The problem with top-down is, that we do not have a set of rules that work under all circumstances and it is hard to agree on such principles. For bottom-up the problem is, that some decisions are very rare and therefore can almost never be observed by an agent and even if it could, people do not always make ethically correct decision due to a lack of information or time (Etzioni, 2017).
Because both have their flaws Amitai and Oren Etzioni (Etzioni, 2017) proposed a hybrid approach, where some rules that are based on the laws are implemented top-down and others such as the personal preferences of the user are implemented in a bottom-up fashion. In order for such a user to exist we need to think of the AI as a partner of the human and not as a fully ethical autonomous entity, which they call AI Mind.
Even if a AI is based on the law there are choices that are seen as ethically better, but often are only followed if everyone obeys that rule. For example, people expect an autonomous car to sacrifice the passengers in order to save more lives, but do not prefer such a rule in their own car (Bonnefon et al., 2016). These scenarios are called social dilemmas. Climate Change can also be seen as such a dilemma, because most people know about the impact of their behaviour on the environment but do act in a way that is undesirable for the collective (Capstick, 2013).
Even if, for example one car manufacturer strictly implemented an agent that works towards the greater good in their autonomous vehicle, people would most likely choose to buy an autonomous vehicle that acts selfishly. Therefore profit-maximizing firms will not care about the deep ethical questions. This shows the need for political answers about ethically correct decisions for agents (Casey, 2017).
Similar to the hybrid approach by Amitai and Oren Etzioni another two step approach was proposed by Dehghani in 2008 (Dehghani et al., 2008). Here they first take the deontological approach to follow some rules and if the rules are not clear the implementation would take a consequentialist view where the best overall outcome is taken. This could overcome the above mentioned problem where people choose a selfish option instead of one that works towards a greater good and would be desirable by themselves.
Reinforcement learning, often based on deep learning, is the state of the art for agents to choose an action. Because they are based on rewards and learn how to optimize for that, a penalty on the reward can be implemented for unethical decisions in a consequentialist way. The deontological and virtue ethics are less popular in the field of AI research, but are those that most likely will be enforced by law and ethical decision frameworks. It is crucial that the law and guidelines can be incorporated into Artificial Intelligence and more research needs to be done to be able to implement given laws.
References
- Hagendorff, T. (2019). The ethics of AI ethics – an evaluation of guidelines. CoRR, abs/1903.03425arXiv 1903.03425. http://arxiv.org/abs/1903.03425
- Himmelreich, J. (2018). Never mind the trolley: The ethics of autonomous vehicles in mundane situations. Ethical Theory and Moral Practice, 21(3), 669–684. https://doi.org/10.1007/s10677-018-9896-4
- Trubia, S., Giuffrè, T., Canale, A., & Severino, A. (2017). Automated vehicle: A review of road safety implications as driver of change.
- Etzioni, A. (2017). Incorporating ethics into artificial intelligence. Springer Science+Business Media, 21. https://doi.org/10.1007/s10892-017-9252-2
- Bonnefon, J.-F., Shariff, A., & Rahwan, I. (2016). The social dilemma of autonomous vehicles. Science, 352. https://doi.org/10.1126/science.aaf2654
- Capstick, S. (2013). Public understanding of climate change as a social dilemma. Sustainability, 5, 3484–3501. https://doi.org/10.3390/su5083484
- Casey, B. (2017). Amoral machines, or: How roboticists can learn to stop worrying and love the law. Northwestern University Law Review, 111, 1347–1366. https://doi.org/10.2139/ssrn.2923040
- Dehghani, M., Tomai, E., & Klenk, M. (2008). An integrated reasoning approach to moral decision-making. https://doi.org/10.1017/CBO9780511978036.024