This article was written for my employee Artifact SA
Mitigating climate change is the most severe challenge humankind has ever faced and reducing energy consumption is one of the critical elements to tackling that problem. Digitalization and data science can have a massive impact by optimizing energy-intensive processes like transportation, where many companies already use algorithms to plan their routes. With more interconnected devices like autonomous vehicles, this will get even more important.
With such use-cases, data science and AI are seen to have an overall positive effect on mitigating climate change. But it is also predicted that ICT will be responsible for over 20% of energy consumption in 2030.
It is often said that data is the new oil, but it is our responsibility that this statement is not true regarding the impact on our climate. Every bit of warming matters, and there is enormous potential to reduce energy in data science.
Data centers
Massive energy is used in data centers, and making them greener is essential to CO2 neutrality. Much energy is used for cooling, and having the data center located in regions where free airflow can be used instead of air conditioning has a huge impact. If that is not possible due to, for example, privacy restrictions, different optimization like utilizing the waste heat can be considered. Such techniques scale better for large data centers and moving the computing to a cloud helps. Having more efficient data centers can even be assisted by AI itself by smart cooling as done by Google. Besides the data center, optimization can be done by limiting unnecessary computations. Already the base operating system needs resources, and containerization and elastic computing can optimize the number of running computer nodes such that unneeded nodes are switched off.
Data usage
On data, some form of elastic usage can also be applied. The vast amount of data stored is hardly ever accessed, and this opens the opportunity to move such cold data to storage that is not always on, like disks that only start if there is access. Such multi-tier storage not only saves money as cheaper storage can be used, but also impacts energy consumption. Inefficient usage of data is another important factor, which can be mitigated. This is often done intuitively because more eco-friendly accessing is mainly correlated with speed. Efficient usage can be achieved by having correct indexing and querying of data. Especially for programs that are executed very frequently, it is worth having a deeper look into the executions. Appropriate data structures and only querying the same data only when needed can be beneficial too.
Efficient training
Training machine learning models often take hours or, in some cases, even months. The famous GPT-3 language model used for training once as much energy as it would need to drive a car to the moon and back. If this is done once and the model gives great benefit, this is a manageable problem. It is worth checking if pre-trained models can be used before thinking about building your own model from scratch. Even if the use case differs, some knowledge about, for example, the language structure is already captured in the language model BERT, which is publicly available and can be tuned for your own dataset (transfer learning). GPT-3 may be an edge-case of a massive model, but if millions of smaller models are trained around the world, these emit quite some CO2. Often a model is not just trained once but hundreds of times for hyperparameter-tuning. Using clever methods like Bayes based optimization, this number can be reduced drastically. Combined with early stopping to detect when it is not worth training further, this can reduce energy consumption and make development faster. To not have to retrain the model multiple times, it is recommended to save the model and reload it for later tests. But often, already low-complexity models that need much fewer resources do a good job and should be considered before taking a sledgehammer to crack a nut.
Inference
Not just training uses much energy; it is estimated that 80% of the energy used for artificial intelligence is for inference. Besides using low-complexity models and optimizing the hardware, there is great potential in new technologies like neuromorphic computing. The human brain operates with only around 15 watts and can do tasks at such a speed that a regular desktop computer using 150 watts would take ages or cannot even achieve it. Brain-inspired hardware with algorithms closely based on how neurons in the brain learn is researched worldwide. Those processors are based on analog signals and do not need to execute every single operation in a neural network on a digital circuit. On the path to such cutting-edge technologies, there are also processors built, which are optimized for executing neural networks and are not just faster but also ecological.
As data scientists, it is our responsibility to ensure that our work is sustainable and ethical. By taking into account the environmental impacts of our practices, and by adopting responsible and efficient methods for collecting, storing, and analyzing data, we can contribute to the creation of a more sustainable future. With this prioritization we can not only mitigate the negative impacts of our work, but also generate new knowledge and solutions that can help address some of the most pressing challenges of our time.
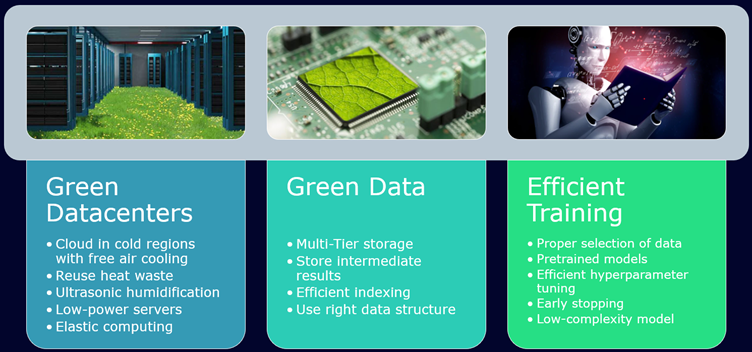